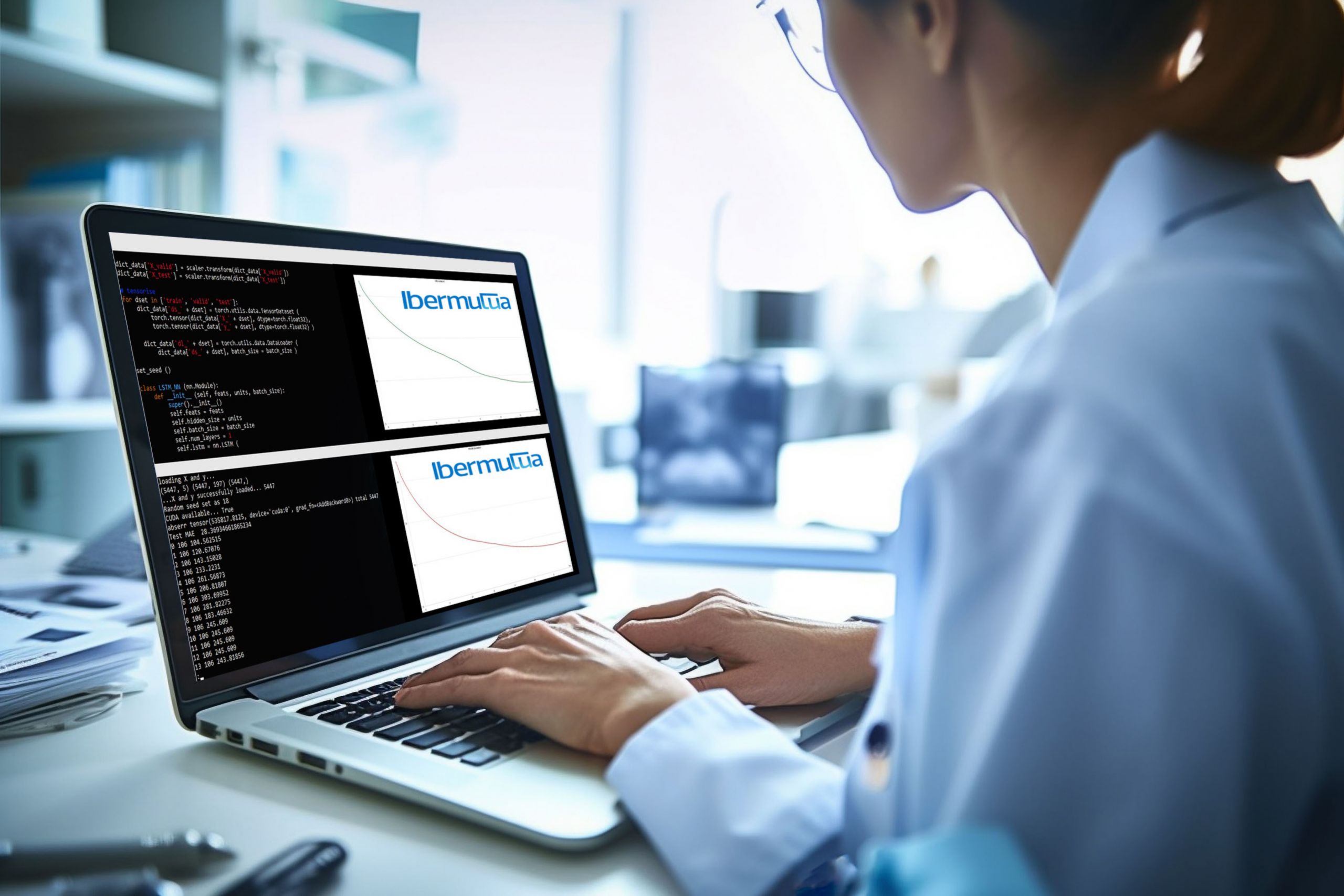
SmartPrognosis: Data of value in healthcare and how the identification and use of key information can improve the management of work-related disability
25 November 2024.
Author(s): Enric Medina Ripoll, Cristina Herrera Ligero, Rosa Mª Servián Carroquino (*), David Corredor Oca (*), Juan Rueda Vicente (*), Helios de Rosario Martínez, Miguel Ángel Lorenzo Agudo (*), Ignacio Bermejo Bosch
Instituto de Biomecánica (IBV)
Data analytics and, in particular, artificial intelligence have the potential to improve the management of work-related disability by providing a prognosis of the evolution of workers on sick leave. However, many AI-based approaches have failed due to the fact that they have been developed from a purely technical perspective. The SmartPrognosis protect has been developed by Ibermutua and the IBV from a clinical perspective, adding to the AI models key information that did not exist in the mutual insurance company’s clinical history. This innovative approach has made it possible to develop applications with high-performance models capable of improving Ibermutua’s management of work-related disability.
INTRODUCTION
In Spain, mutual insurance companies that collaborate with the Social Security (hereinafter referred to as MCSS), such as Ibermutua, are entities that manage various economic benefits and services related to the occupational health and safety of affiliated workers and companies.
The most significant benefits associated with absenteeism that they currently manage are those arising from temporary disability (TD) and permanent disability (PD). In this respect, MCSS alone manage more than 7 million cases each year, generating benefit payments in excess of 10 billion euros.
Given such astronomical figures, effective case management not only contributes to the financial sustainability of the social security system, but also ensures that workers can enjoy a better quality of life. By identifying and prioritising the cases that have the greatest economic and social impact, MCSS can implement early intervention strategies that help to reduce the duration of sick leave and optimise available resources. This translates into significant savings for the system and a quicker and more effective recovery for the worker.
Providing tools and procedures that make it possible to make the best decision for each specific patient, considering their individual context and characteristics, therefore has a direct impact on their quality of life and on the sustainability of the social security system. This approach is in line with the value-based medicine philosophy, where the measurement of any intervention takes into account both classical clinical indicators, such as the patient’s context and preferences, as well as efficiency in the management of resources or the costs incurred.
DEVELOPMENT
The use of data in healthcare: a new opportunity driven by artificial intelligence
The scientific community supports personalised medicine as a game-changing alternative that will improve the diagnosis and treatment of patients. This holistic approach involves the use of multifactorial information – clinical data, genetic predisposition, complementary tests, habits, patients’ perspectives and perceptions, and information related to their daily lives – to tailor interventions to each person and their context. However, how they are implemented in the real world is more of a grey area. Clinicians often face the challenge of having to work with incomplete or biased information. This situation makes it difficult to identify and manage more complex cases and to choose the most appropriate treatment. This is largely due to the lack of structured secondary information on “non-clinical” determinants (cultural and socio-economic factors, life experiences, etc.) that also impact on health and influence the resolution of cases.
The digitisation of health data has transformed how medical information is accessed and used. The availability of digital data facilitates access to complete medical histories, records of previous treatments and results of interventions, providing an evolving overview of the patient. While this is an advantage, the availability of structured secondary information related to non-clinical health determinants remains scarce, making it difficult to implement truly person- and patient-centred approaches, an aspect that is increasingly relevant for health systems themselves.
In any case, access to these large amounts of information provides an opportunity to apply new data analysis strategies based on artificial intelligence (AI). In short, if we are able to analyse all the information that affects the patient’s evolution, we will not only achieve a diagnostic impression that is closer to reality, but we will also avoid the biases that result from making a decision based on incomplete information.
The benefits of this approach are plain to see: improved accuracy in identifying pathologies, optimisation of treatment plans and prevention of complications; in short, reduced process times and more efficient management of resources, while providing added value to healthcare staff and systems.
Requirements for a tool to improve the management of temporary work-related disability
In the specific context of the management of temporary work-related disability, the identification of key information in care processes and the development of tools based on artificial intelligence that enable the targeted analysis of this information, can help to identify, at an early stage, those processes that have a greater impact in terms of costs and recovery times, and those that offer significant opportunities for improvement.
Such tools can facilitate evidence-based decision making, achieve greater accuracy when identifying cases that have such an impact, and optimise intervention strategies, directly influencing the quality of patient care and reducing costs. In short, a solution of this nature would add value by contributing to the sustainability and efficiency of the Spanish welfare system and improving the recovery of workers.
However, developing solutions from a purely mathematical perspective is a guarantee of failure, which is why, before any development begins, we need to have a thorough understanding of the map of the healthcare, administrative and medico-legal processes, and identify the key information for the resolution and prognosis of the processes and their availability. This is a highly complex framework, and gaining such a thorough understanding of it requires the contribution of multidisciplinary teams that include healthcare, administrative, technical and legal profiles, and the application of the most appropriate analytical techniques, particularly those based on user experience design (UX).
Another key issue is the need to focus on improving the management of temporary work-related disability. An AI model that predicts the duration of a sick leave does not, by itself, improve the quality of care or optimise the use of resources. This is one of the reasons why it is essential to understand how MCSS work and in which situations their interventions can improve a patient’s quality of life and/or save resources. AI models will therefore be useful to the extent that they answer specific questions that have been identified as relevant to being able to improve the performance of healthcare and financial resources, for example: Which patients are the most complex and deserve the most attention? Which intervention is likely to be most successful for a given patient? Which patients are most likely to return to work early?
In view of the challenges and opportunities identified, Ibermutua promoted the development of the SmartPrognosis project, with the IBV as a strategic partner.
SmartPrognosis: Can sick leave management be improved by an analysis of prognostic information?
The main objective of the SmartPrognosis project is to develop a tool to improve the management of temporary work-related disability at Ibermutua, both for work-related and non-work-related pathologies. On a technical level, this objective translates into the identification and processing of the key information found in clinical histories, the identification and recording of currently unavailable prognostic information, and the development of a model based on artificial intelligence that predicts the duration of the temporary work-related disability process. Another important element of the project is the study of the duration of a case depending on the characteristics of the patient and the alternatives available.
Implementing this solution involves having to overcome a number of technical and ethical challenges, including protecting workers’ personal data and ensuring the fairness of the predictions. Another crucial factor is the cooperation of the mutual insurance company at every level: medical, technical and management.
In this regard, in order to develop an AI model that provides the mutual insurance company with information on the duration of the case and helps it to manage the case, it was essential to address several key aspects:
- Alignment with the mutual insurance company’s processes and objectives: The model had to take into account the specific processes and strategic objectives of the mutual insurance company. This implies the need to understand that MCSS do not act as standard health centres, but rather have a broader focus that includes the management of financial benefits for temporary and permanent work-related disability, among others, as well as healthcare related to work-related sick leave. The model should therefore help to achieve these objectives.
- Identifying and collecting relevant information: In order to make accurate prognoses, it is essential to identify what information is needed and how it can be obtained. This includes detailed clinical data, employment records, information about the work environment and any other factors that may have a bearing on the worker’s recovery. Procedures must also be put in place to collect whatever information is missing, which may require collaboration between the MCSS, the employers and the health professionals. Data quality and integrity are critical to the accuracy of any model.
- Preparing models to answer key questions: The development of the model had to be guided by key questions that address the specific management challenges faced by mutual insurance companies. This meant that the model had to be able not only to forecast the duration of the temporary disability (TD), but also to identify cases that could benefit from early or personalised intervention, to assess the risk of transition to permanent disability (PD), or to suggest different approaches from a medical and administrative point of view. Ultimately, it is the model’s ability to provide actionable and relevant answers that will improve the said management.
- Combining clinical and technical expertise: The effectiveness of such a model depends on close collaboration between clinical and technical experts. This collaboration between healthcare professionals and AI experts ensured that the eventual solution would be clinically relevant and technically sound.
- Compliance with current regulations: Any AI-based solution that was developed had to comply with applicable local and international regulations. This included ensuring data security, efficacy and privacy, as well as obtaining the necessary approvals prior to implementation. Regulatory compliance is not only a legal obligation, but also ensures the security of the solution and instils the necessary confidence in clinical staff and employees.
Therefore, a solution such as the one proposed in the SmartPrognosis project required a comprehensive approach that combined technical and clinical expertise, an approach based on the specific needs of the mutual insurance company, and a commitment to data quality and regulatory compliance.
The SmartPrognosis work plan
A four-phase work plan was designed to ensure that the defined objective and requirements could be achieved (Figure 1):
Figure 1: Work Plan
PHASE 1: Selection of priority cases
As each condition or pathology has a number of specific characteristics that imply differences in the map of the associated care case, it is important to characterise each case individually. In the context of the SmartPrognosis project, we began working on pathologies that have a particular impact on mutual insurance companies due to their prevalence or complexity: work-related shoulder pathology (OCC) and non-work-related spinal pathology (CC).
PHASE 2: Identification and collection of relevant data
As mentioned above, it is essential to identify what information is of real value in terms of solving the problem we need to answer, and to understand its significance. This includes variables that are available in the clinical history and others that have been identified as valuable but are not currently collected, or at least not in a structured way. Without key information, AI-based analytic models will not fulfil the desired function because they are not based on data with real prognostic weight.
To accomplish this task, it is essential to understand both the clinical language and how to apply the most appropriate research techniques to identify additional valuable information. Phase 2 was therefore divided into three sub-phases:
● Phase 2a: A study of the data included in the patient’s clinical history.
● Phase 2b: Identification of new data relevant to the care case but not currently recorded as standard in clinical histories. To this end, we carried out an initial review of the scientific literature and, in order to characterise the care case map, we visited the mutual insurance company itself, using a specific research methodology based on the Delphi method, including interviews, discussion groups and surveys of key agents sufficiently representative of the mutual insurance company (a total of approximately 100 professionals).
● Phase 2c: A pilot study to obtain and add the data identified as being of prognostic value to the patient’s clinical history. The pilot study allowed us to add new valuable data to improve patient prognosis. This pilot phase ran from February to November 2023. 1,616 patients and 315 doctors agreed to participate in the study, and the required information was finally completed in 507 cases.
PHASE 3: Development and validation.
Having identified the relevant data, we then defined the neural network architecture for the development of a prognostic model for the duration of care cases. Figure 2 shows the block diagram of the network used.
Figura 2: General diagram of the SmartPrognosis model
The model consists of three blocks:
- Block 1 Static branch: The branch responsible for analysing patient data.
- Block 2 Temporal branch: This branch of the network consists of recurrent neurons and is responsible for processing temporal information (episodic data).
- Block 3 Top model: The outputs of the previous branches are concatenated into a single data vector which is then used as input to the final stage of the network. This branch provides the output of the model: number of days off work.
The neural network was prepared by using 214,711 episodes taken from the Ibermutua clinical history (70% of the database) as the “training dataset”, plus another 61,346 episodes (20% of the database) as the “validation set”, which was used during the training to assess the network’s ability to generalise with new cases, and to adjust the training hyper-parameters. The remaining 10% of cases (30,674) were used as a “test set” after training had been completed, to independently assess the accuracy of the neural network.
The validations performed during the project made it possible to conclude that the model performs well. One of these validations was the comparison between the number of days of sick leave estimated by the clinical professional of the mutual insurance company or the public health service when issuing sick leave and the duration of sick leave estimated by the model. Figure 3 shows that the cumulative failure total (equivalent to the sum of the total number of days of error between the estimated duration and the actual duration at each point and at the previous consultation points) is 2.5 times greater in the clinical prognosis than in the model prognosis. This result confirms that the model we have developed is better at predicting the duration of the case than a prognosis based solely on the opinion of the healthcare professionals. Therefore, on the basis of this model, which takes valuable information into account, and by specifically adapting the model to the contexts of use defined by the mutual insurance company, we can have a tool that improves the management of resources and allows substantial savings in terms of days off work.
Figure 3: Error in prediction of TD days based on clinical opinion and based on SmartPrognosis
Phase 4: Commissioning and next steps
In parallel with the fine-tuning of the model, work was carried out to define the specific contexts of use of the solution in order to improve the management of temporary work-related disability at Ibermutua. The following contexts of use are of particular importance:
● Application 1: Identification of episodes in which patients may be able to return to work in a short period of time and in which it may be worthwhile to intervene in order to speed up the process.
● Application 2: Support for medical-administrative decision making by identifying different case durations according to the proposed approach.
● Application 3: Identification of cases where the process to reach a considered diagnosis is likely to take longer, in order to manage the allocation of resources and/or monitoring and to make decisions that are more appropriate to the case.
An implementation procedure is currently being designed to enable Ibermutua to evaluate the real impact of the solution on management in terms of worker satisfaction, healthcare professional satisfaction and improvement in management indicators.
Example of implementation in the identification of cases with a likelihood of a rapid return to work “return to work cases” (Application 1)
One of the potential contexts of use of SmartPrognosis is the usefulness of the model in the identification of discharge episodes in a short period of time.
In this context, the model provides a list of patients ordered by highest probability of proximate discharge (Figure 4). In this way, the clinicians responsible for the follow-up of hundreds of cases of common contingency would have a tool that would allow them to prioritise the appointment of those workers in whom it is more convenient to assess a discharge from work, which would result in a better use of the health resources of the mutual insurance company.
Figure 4: Diagram showing how SmartPrognosis provides an ordered list of patients with a high likelihood of a rapid return to work, allowing clinicians to prioritise the review of these cases.
Figure 5 shows one of the validations performed on one of the lists of patients with the highest probability of discharge. The bars in the figure indicate the order of prioritisation of the entire sample of listed patients. In the ideal situation (first column), only ‘dischargeable’ patients (green) should be cited. However, in the usual situation (second column), some of the patients cited deserve to be discharged (green colour) and others, on the other hand, need to continue in IT (orange colour), wasting human resources on citations that do not contribute to the process. With the use of SmartPrognosis (third column), which proposes a prioritisation of patients, the model is able to detect a large number of ‘dischargeable’ patients, and the hit rate of ‘dischargeable’ patients is very close to 100% in the first prioritisation groups.
In this sense, this tool allows us to approach an ideal situation, which will allow clinicians who manage temporary disability processes derived from common contingencies to focus their efforts on cases that really require attention and where there is a possibility of discharge, thus significantly improving the efficiency of the organisation.
Figure 5:List of the prioritisation of the 338 patients assessed. The ideal prioritisation, the usual prioritisation and the prioritisation based on SmartPrognosis are shown
This application will enable clinicians managing temporary work-related disability processes arising from non-work-related cases to focus their efforts on cases that really need their attention and on those where the patient is likely to be able to return to work, this improving the efficiency of the company.
Impact of adding new clinical and contextual data
Within the framework of the SmartPrognosis project, we have added several of data to the clinical history that were not previously included: patient context, clinical, perception and preference data. These data have made it possible to develop advanced models that increase the accuracy of the disability prognosis, especially at the beginning of the process.
To evaluate the impact of the experimental data on the improvement of the model, we carried out two types of studies.
On the one hand, an analysis of the variance, as explained above, in the duration of the temporary disability (TD) in all the variables used in the model. This work led us to conclude that the data obtained in the experimental phase have the greatest impact on the duration of a TD, followed by the type of contingency variable (whether the case is work-related or non-work-related).
On the other hand, we compared the results obtained by the model based purely on medical history data with the model with added data from the experimental phase in the second week of evolution. The study concluded that the model with experimental data improves the accuracy of the prognosis in some of the applications by 13% compared to the model based purely on a patient’s clinical history.
CONCLUSIONS
Thanks to artificial intelligence and data analytics in general, it is possible to analyse large amounts of data from different sources, and thus contribute to the development of the concept of personalised medicine. However, many initiatives related to data analytics have failed because they have been developed exclusively from a purely mathematical perspective, which is why the SmartPrognosis project has prioritised improving the health management of temporary work-related disability over any other technical aspect – a pioneering and sadly rare approach in the field of health technology. In the case of the SmartPrognosis project, the main focus in the development of such a technological solution has been on the clinical profile, reflecting the importance of a thorough understanding of healthcare processes, the relevance of clinical data, and the importance of recording valuable new information on a case-by-case basis. This strategy has made it possible to generate a solution designed to solve specific problems, taking into account the specific conditions of the mutual insurance company.
The SmartPrognosis project has also shown that certain data relevant to understanding the impact of social and health interventions are not always taken into account. Hence, before starting to develop any mathematical model, it is essential to identify the key information needed to solve the target problem and, if necessary, to implement a methodology to collect valuable data related to the patient’s context that is adapted to the characteristics of the entity.
The model developed in the SmartPrognosis project has been shown to be more accurate than the prognosis proposed by healthcare professionals, probably due to the fact that less information is available to the latter when making the prediction, with incomplete or biased data in many cases.
The first applications we have developed show that the tool can help to improve efficiency in the use of healthcare resources, allowing us to focus on the processes that really merit attention.
The next steps in the project will be to further improve the predictive power of the model, both through possible new analytical approaches and by supplementing the available information with data related to the patient’s context, always identifying and prioritising those with the greatest prognostic value. The next objective is to implement the models in the selected processes and evaluate their real impact on management.
AUTHOR’S AFFILIATION
Instituto de Biomecánica de Valencia
Universitat Politècnica de València
Edificio 9C. Camino de Vera s/n
(46022) Valencia. Spain
*Ibermutua
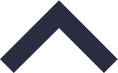